This guideline addresses the challenges of AI adoption in business, providing a framework for responsible implementation. It outlines key concepts, potential risks, and best practices for AI integration. Developed by industry experts, it offers CxOs essential questions to consider and strategies to ensure ethical, transparent, and effective AI use while maximizing business growth potential.

Image credit: Jérémy Barande
Introduction
In March 2024, a collective of business leaders, legal experts, computer and data scientists, marketers and investors convened to address the challenges surrounding AI adoption in businesses. This assembly recognized the growing confusion in the market, instances of AI gone awry and proliferation of ungoverned AI solutions. Consequently the decision was made to take proactive measures and develop an open guideline for the responsible use of AI in business.
We are deeply rooted in the belief that publishing open standards and guidelines accelerates adoption by fostering collaboration and innovation. History has shown that they make it easier to work with third party vendors, integrate applications and foster innovation. As a pioneer in the field of growth acceleration through technology, Touchpoint Strategies has initiated the development of these guidelines.
We are committed to guiding businesses in leveraging AI ethically for growth. These guidelines are written to give business leaders an understanding of AI, how it can benefit their companies, what can go wrong in the use of AI and empower them with the key questions to consider.
Our team at Touchpoint Strategies brings a human touch to the complex world of AI implementation. We understand the challenges businesses face in adopting new technologies, and our commitment extends beyond mere consultancy. We partner with our clients every step of the way to ensure their success. In 90 days we help clients identify areas of growth with high ROI and show them how to use technology to deliver that growth.
The Basic Concepts
As Andrej Karpathy wrote in his seminal article Software 2.0, AI is fundamentally different from other kinds of software in that, “No human is involved in writing this code.” Instead, AI learns its “code” on its own from data. That’s why AI needs more governance and oversight than traditional software:
AI applications have little human oversight during development and thus need more oversight during operations.
Here are some general definitions to work with. “Artificial Intelligence” (AI) is software that is able to perform tasks that normally require human intelligence, such as visually identifying objects, speech recognition, financial decision making, writing computer code, and translating between natural languages such as English and French. Some of its most widely used applications are internet search at Google and Bing, product recommendations at Amazon.com and Netflix, and news feeds at Facebook, TikTok and YouTube.
“Machine Learning” is a subset of AI that focuses on the algorithms for learning from data, and deep learning is a subset of machine learning that uses multiple interconnected layers of neural networks to learn. Advances in deep learning around 2007 are what led to rapid advances in speech recognition, image recognition, and chatbots like ChatGPT that we have today. Today the best chess and Go players in the world are deep learning programs.
What makes AI so potentially valuable to businesses is that it learns hundreds of millions of times faster than people and previous statistical methods. In 2017, a Google AI program called AlphaZero chess taught itself to play chess by playing against versions of itself millions of times. In just two hours it was better than any human. Since then AI has generated value in most industries including aircraft engine design, food science, and biology.
The Road To AI-Enabled Growth Is Fraught With Challenges
Most businesses still find it challenging to generate significant positive ROI from AI. Common barriers are:
Inadequate Data Management: Many organizations lack the infrastructure for effective use of AI due to low data availability, access, and quality.
Skill Shortages: A shortage of expertise in AI development, deployment, and operations poses a significant obstacle to adoption.
Evolving Regulatory Landscapes: The dynamic nature of AI governance requires businesses to stay abreast of changing regulations and compliance standards.
AI Can Solve So Many Business Issues: The broadness of AI applications makes it challenging to determine the path with the highest potential business ROI. In their 2023 State of AI report, McKinsey reported that the most common challenge to capturing AI value is the lack of a strategy.
Transparency: Treating AI as a black box increases risks. Companies need methods of understanding AI programs so that managers can make informed risk/reward decisions.
Lack of Responsible AI Standards: The Touchpoint Strategies guidelines embodies a commitment to ethical principles, accountability, and transparency.
AI Gone Awry
In the network economy, a company can destroy its brand in seconds and not know that it’s happening until it’s too late. Some brands can regain lost trust but most never will. Here are a few examples of AI risk:
Hospital admissions: A commonly used hospital admissions algorithm erroneously used cost as a proxy for sickness and created a major racial bias. The result was that the algorithm flagged only half of very sick Black patients correctly, resulting in them getting less care for the same level of sickness as other patients.
Home value estimates: The popular real estate website Zillow displayed the estimated value of all homes rather than just those that are listed for sale or recently sold. The company became so confident in their estimates that they began buying 3,000 homes a month that they thought were underpriced. In order to scale further, they did a human review of each purchase. The lack of oversight resulted in Zillow losing $570 million and firing 2,000 workers.
Grocery store price optimization: An AI pricing algorithm at the Canadian grocery Loblaw focused on near-term profits without a sufficient understanding of the dynamics of the grocery market. Thus it raised the price on weekly loss-leaders that loyal shoppers enjoy. As a result they lost market share and about $10 million in one quarter, including over $1 million in computational costs to train the errant algorithm.
Race-changing images: Google found itself in hot water after Gemini — the tech giant's chatbot — generated images of humans from a wide variety of periods and societies that weren’t ethnically accurate. Perhaps the most offensive were people of color as Nazi soldiers. Google paused the tool and CEO Sundar Pichai told employees that “some of [Gemini's] responses have offended our users and shown bias. To be clear, that’s completely unacceptable and we got it wrong.”
Airline chatbot lies about refund policy: Air Canada lost a court case after its chatbots lied about policies relating to discounts for bereaved families. The airline's chatbot told a customer that they could retroactively apply for a last-minute funeral travel discount, which is at odds with Air Canada policy that refunds cannot be claimed for trips that have already been taken. Air Canada's unsuccessful defense was that the chatbot, not the company, was liable, and that they could not be held responsible for the tool’s AI-generated outputs.
Intentional human misuse: The US Securities and Exchange Commission charged Delphia and Global Predictions with breaking regulatory marketing rules by “AI washing.” The companies falsely claimed they were using AI to make smart investment decisions. SEC Chair Gary Gensler said, “We’ve seen time and again that when new technologies come along, they can create buzz from investors as well as false claims by those purporting to use those new technologies. Investment advisers should not mislead the public by saying they are using an AI model when they are not. Such AI washing hurts investors.” The two companies agreed to settle and pay a total of $400,000 in penalties.
Touchpoint Strategies Guidelines For Responsible AI In Business
Elements Of “Responsible AI”
The definition we have applied for Responsible AI aligns with the most common regulatory practices to date and is expanded to apply to business use. Responsible AI is a commitment:
to maintain human oversight over all AI applications and ensure accountability for AI outputs
to adhere to current data and privacy laws and regulations in all geographies where the company operates, plans to operate, or reasonably believes they will conduct business in
to appoint a Chief AI Officer (CAIO), an individual with the necessary expertise and authority to be responsible for the company's AI strategy including AI content and systems, and materials created by AI. The CAIO will oversee the use of data by the systems, train AI applications, and ensure compliance with data governance and management requirements. If internal expertise is lacking, this role may be filled by a qualified paid external party
to clearly define the data and AI biases that are acceptable and how to measure them
to frequently measure the accuracy and biases of training data and AI systems, and take action when they exceed established thresholds
to stay informed about AI governance and laws, comprehend the risks of each AI tool in use, review the licenses of these tools regularly, and understand their potential risks to the business
to lead the creation and maintenance of an AI Employee Training Program and AI Internal Use Policy for employees
Just as businesses have different cultures, they also have different AI bias goals. Some biases are commonly avoided due to regulations such as race and gender biases in hiring. Others are more subjective. For example, a women's apparel retailer might not care that the accuracy of their product recommendations is higher for women than men, or that it tends to suggest higher priced items to men.
Here are the questions to ask yourself and consider before approving AI experiments or the deployment of AI in your operations.
Business
What business problem are you trying to solve and what is the genesis of the problem? Is the use of AI proportional to that problem?
What positive ROI do you need and on what timeline?
What business outcome are you trying to achieve?
What business objective is driving the interest in using AI?
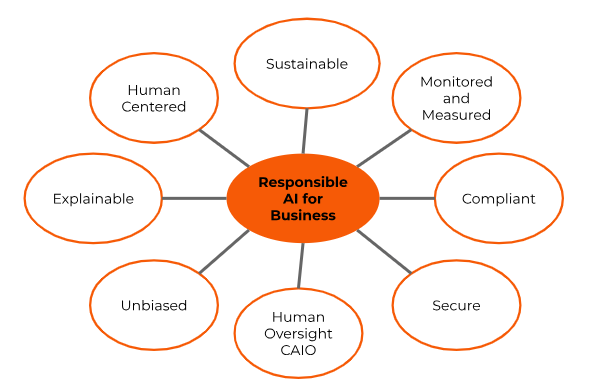
Components of a responsible AI framework
Who is accountable for accuracy and bias?
What systems and processes will AI replace?
Have you effectively evaluated the risk if your AI implementation goes wrong?
Expertise
Does anyone on your team understand your company’s data, its origin and the rules that govern that data?
What data and privacy rules are applicable such as HIPAA, CAN-SPAM, GDPR, CPRA or copyrights?
Do you know the privacy and security of the AI systems you use? Who has read and comprehends external AI platform usage agreements? What Indemnification does the platform provider supply? For example, does your video conference transcription service conform to your privacy policies?
Data
What data collection will occur using an AI solution?
How are biases in key datasets monitored?
Who is accountable for bias violations?
Brand and Culture
What is the sentiment toward AI among your industry, customers, employees, and board of directors?
Who in your company harbors skepticism about AI and why?
Some AI best practices
What we don’t measure does not exist. Define performance metrics that demonstrate a system is performing responsibly and effectively. Don’t “wait and see what we get.”
Success measures: Define clear, measurable goals for each AI application such as improved client engagement, reduced operational costs, or enhanced decision-making accuracy. Work together with users to design an experiment or A/B/n test to measure success before the application is deployed.
Ethical Audits: Regularly review AI applications with member-cross organization, red teams, or outside third party experts to identify and report any ethical concerns, biases, or unintended consequences that could occur. These audits will help maintain a record of testing for ethical AI use.
Iterative Improvement: Publish insights from performance metrics and ethical audits to inform the whole company and ensure AI applications performance in a safe and tested fashion.
Publish New Measures: As your business evolves into being AI powered you will need new KPIs, new approaches to value creation, and ultimately business success.
Invest in change management. You will need to help all employees about AI, understand its impact on the company, the customer, and their individual job. Failing to do so puts AI ROI at risk. The history of AI is riddled with case studies of accurate, ethical systems that never were used.
Accept that there will be failures and reward finding them early.
Performance: Don’t try a moonshot for your first implementation and don’t pick too simple a task like AI driven subject lines in our outbound emails. Find a legitimate business challenge that can take you towards your blue ocean of growth.
In Summary
These Responsible AI guidelines are designed to help businesses effectively implement and adopt AI.
By engaging internal and external stakeholders
Through transparency in your data, toolsets, AI outputs
By considering additional ethical and legal factors
By asking good questions, considering risks and making human-centered choices
Comments